In analyzing long-read RNA-sequencing data, defining a custom transcriptome by identifying novel isoforms is a complex yet essential task that can have far-reaching consequences for any downstream analyses. As long reads allow researchers to examine isoform diversity in significantly greater detail than short reads, long-read sequencing often reveals a large variety of isoforms not yet present in reference annotations, even for model organisms.
Though error rates in platforms such as Oxford Nanopore or Pacific Biosciences are steadily decreasing, the separation of true novelty from biological or technical artifacts remains a challenging problem, with many computational tools utilizing a variety of strategies to address this.
Different experimental conditions, such as tissue, sex, or disease, can exhibit notable differences in isoform diversity. One common approach to isoform identification for experiments with multiple samples is to pool the reads from all samples to define a unified transcriptome. While this approach can be beneficial, e.g. by combining evidence for rarely occurring isoforms from multiple samples, it can also be computationally costly as well as possibly miss rarely occurring sample-specific isoforms.
To address this issue, another approach is to identify novel isoforms separately for each condition, e.g. tissue, to ensure that even rarely-occurring and sample-specific isoforms are identified. To then combine such condition-specific transcriptomes into a single, unified transcriptome suitable for further analysis, OmicsBox now offers the tool TAMA Merge.
How to use TAMA Merge in OmicsBox
Starting from the same long-read RNA-sequencing data of brown bears as was used in the recent blog post “End-to-End Analysis of Long Reads in OmicsBox”, FLAIR can define an individual transcriptome for each tissue type: adipose, skeletal muscle, and liver. Once FLAIR has identified novel isoforms in each tissue, including those that may be specific to only one of the tissue types, TAMA Merge can combine these tissue-specific transcriptomes to form an overall brown bear transcriptome for further analysis.
In OmicsBox, TAMA Merge is located in the Transcriptomics Module, under Long-Read Analysis, and Combining Transcriptomes with TAMA Merge.
Inputs
The input consists of the sample-specific transcriptomes, as well as the publicly available reference transcriptome. Choosing to prioritize the reference transcriptome conserves the splice junctions as well as transcriptions start and end sites of reference transcripts. Furthermore, it transfers transcript IDs from the reference to the output, where available.
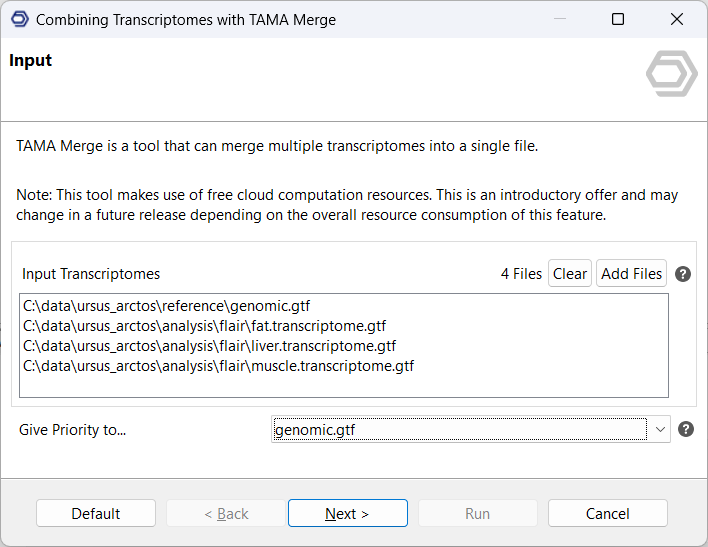
Algorithm configuration
The next page defines the algorithm parameters. First, the option “Capped” determines whether the transcription end sites are trustworthy, or whether it should always merge shorter transcripts into longer ones. As FLAIR implements its logic on how to define a transcript, “Capped” is suitable for this analysis. The option “Exon Ends” determines whether the algorithm will choose the most commonly occurring, or the longest exons when merging transcripts with different start- and/or end sites.
Perhaps the most important settings are the thresholds for merging transcription start and end sites, as well as splice junctions. One useful approach is to set the splice junction threshold to 0 while setting the thresholds for transcription start and end sites to 50. This means that transcripts can only be merged when their splice junctions are exactly at the same position, whereas for start and end sites, there is more tolerance. The reasoning for these settings is that start and end sites can exhibit significantly more variation e.g. through degradation of RNA, but this variation does not always accurately represent different transcripts.
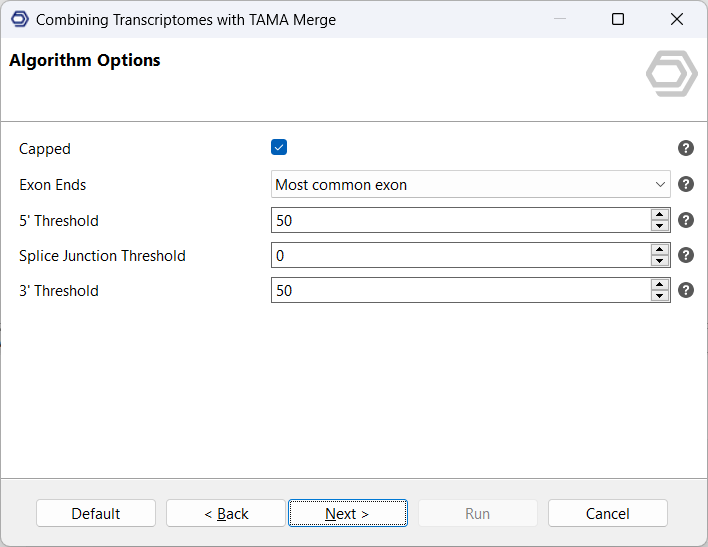
Outputs
In addition to the merged transcriptome in BED and/or GTF format, TAMA Merge also generates:
- a Merge Report, which maps transcript IDs of input files to the resulting merged transcripts;
- a Gene Report, which contains information about each gene, including how many transcripts it had before and after the merge;
- a Transcript Report which contains information about each transcript, including which source transcripts were merged to create it.
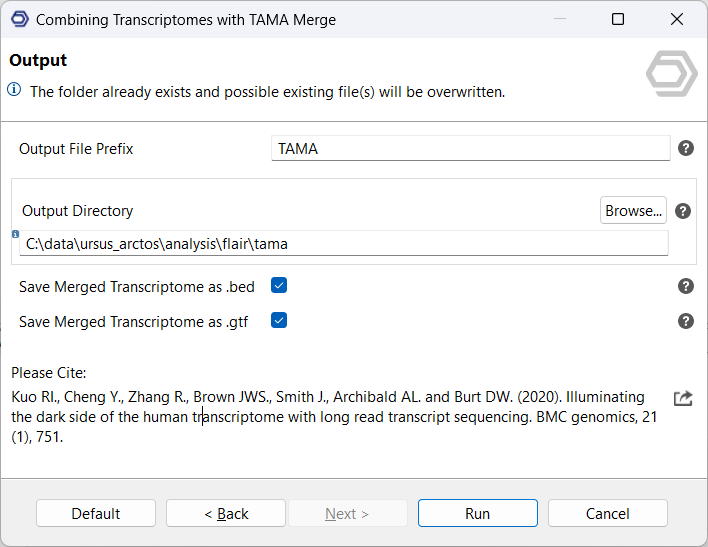
Investigating the results
To get a clearer picture of the results of TAMA Merge, the input and output transcriptomes can be visualized together in the OmicsBox Genome Browser.
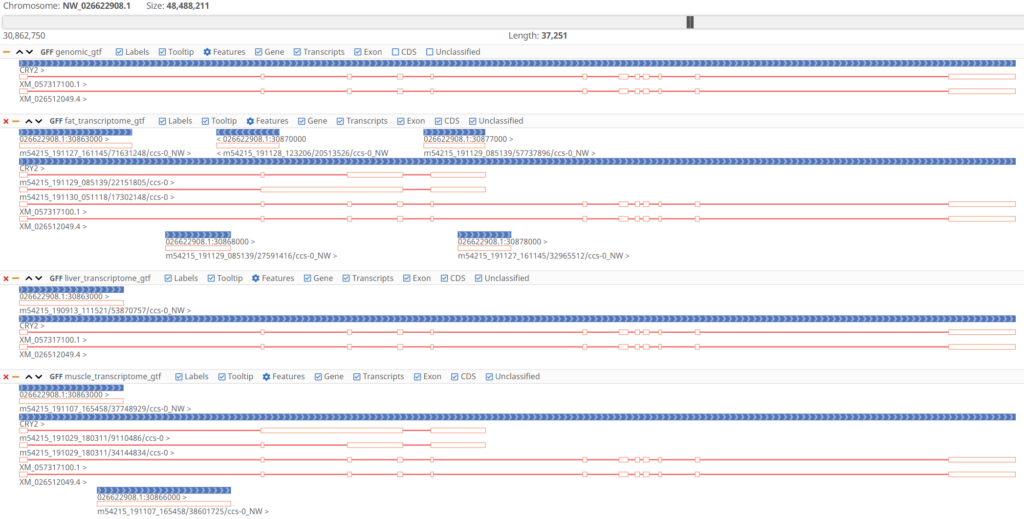
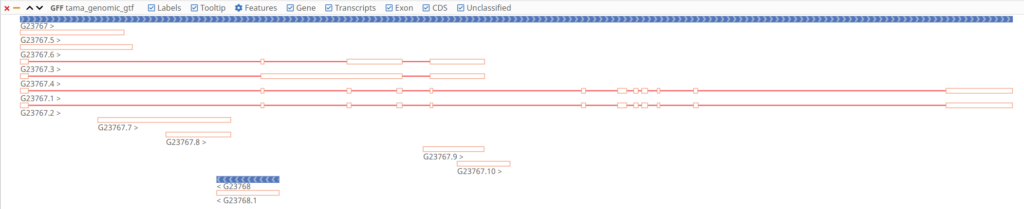
Curating the transcriptome
Finally, SQANTI3 can characterize and curate the merged transcriptome. This not only provides a more detailed view of the isoform diversity but also ensures that subsequent analyses only consider trustworthy, high-quality transcript models.
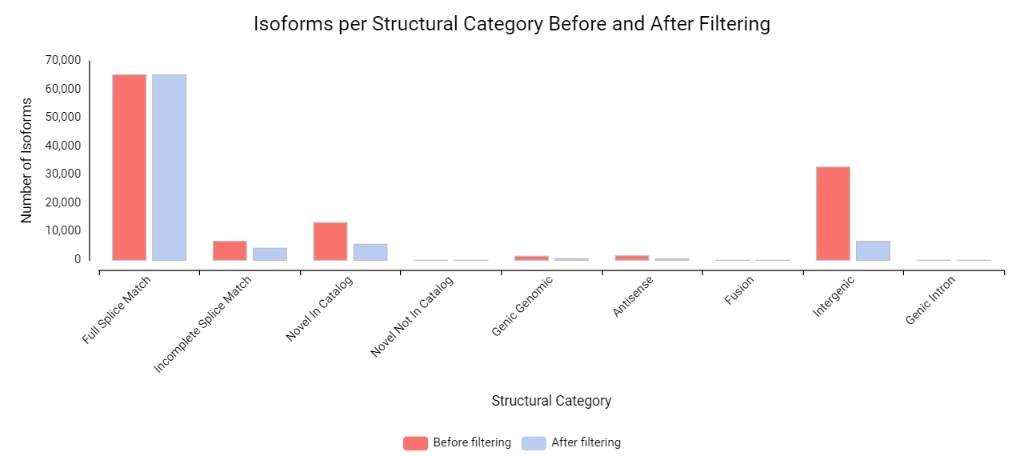
Conclusion
The workflow of FLAIR, TAMA Merge, and SQANTI3 within OmicsBox can identify even rarely occurring isoforms that are specific to experimental conditions and subsequently merge them into a curated, high-quality transcriptome for further analysis. This can potentially enable novel discoveries in downstream analysis by uncovering subtle differences between experimental conditions.
With the inclusion of TAMA Merge, OmicsBox continues to expand its repertoire of user-friendly end-to-end analysis workflows, which includes the growing collection of tools aimed at long-read data analysis. Together with our collaborators, BioBam is also contributing to some exciting research regarding long-read data analysis, and we hope to be able to share some of the results of these efforts with the community soon!
References
Kuo, Richard I., et al. “Illuminating the dark side of the human transcriptome with long read transcript sequencing.” BMC Genomics 21 (2020): 1-22. https://doi.org/10.1186/s12864-020-07123-7
Tang, Alison D., et al. “Full-length transcript characterization of SF3B1 mutation in chronic lymphocytic leukemia reveals downregulation of retained introns.” Nature Communications 11.1 (2020): 1438. https://doi.org/10.1038/s41467-020-15171-6
About the Author